$100m AI Product Leader’s Checklist: 5 Key Criteria to Validate Your Next Big AI Idea
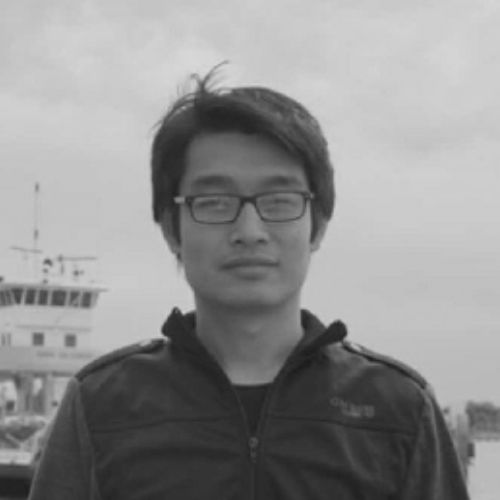

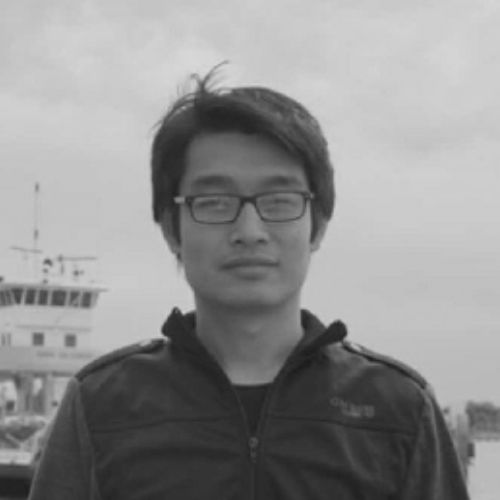
Over the years working on various AI products and technologies at top American tech giants, I have unconsciously formed a checklist in my mind for evaluating whether an AI product direction is promising. These are principles and standards for checking if an AI product direction is good. And many of them are about what “not to do”.
Clearly defining what not to do is often more important than defining what to do. Setting boundaries and constraints can actually make things easier.
I have summarized a few criteria that I use to evaluate ideas:
1. Is this “new”? Does it rely on recent AI advances to work?
This wave of AI is driven by breakthroughs in large scale training and generative models. So first judge if your direction is new — whether it can do things not possible before, enabled by recent LLMs. This gives it meaning.
It makes no sense now to work on old AI directions — classification models, script-based dialog systems, intent-based chatbots. These don’t work, as proven over past years.
Of course this requires deep understanding of the latest AI tech and models, the core innovations and breakthroughs compared to past years. This needs grasp of models, ML, statistics, and hands-on experience.
2. Does this product save costs or enable new capabilities?
Cost saver or enabler? I don’t do cost savers. Just reducing costs has no future. Although media hypes AI replacing jobs and cutting costs, simple automation has little value. Hard to sell.
Driving change is far harder than you imagine. Scientific Advertising argues that changing habits is extremely expensive. Don’t try to change people’s habits — even valuable products like new toothbrushing methods won’t make money, because 20% cost savings isn’t worth changing workflows.
Prioritize enablers — make the impossible possible. Many opportunities here need deep thinking beyond recent AI.
3. How high a success rate does your AI product need?
An easily overlooked detail.
AI is hyped as amazing, but you may not realize success rates are often not that high. Maybe 90%, but more like 70–80%. Use it 10 times, works well 7–8 times, stupid otherwise. And you can’t tell which times it will work. Lots of manual reviewing needed.
When people boast about AI, they imagine it working perfectly, not considering 20% failure cases. Users seeing a dumb AI.
Consider AI failure impacts on users. How will they view your product? Effort to check and correct AI results?
Find cases where dumb AI has low user cost — like TikTok recommendations. Often wrong, but users just swipe away, barely notice.
Contrast this with self-driving cars — even 99.9% accuracy has catastrophic 0.1% failures.
Users mentally judge likely AI success before using a product, and whether it’s worth their time. Low perceived accuracy means no users. People are lazy today, their time is precious.
Note this is about users’ mental models of your AI accuracy, not actual performance. Why conversational AI is hard — users assume it will fail and just switch to human agents, not even trying it. Cognition shapes this.
4. What the real long term moat?
Some valuable areas have no defensibility so I avoid them. AI platforms like OpenAI have no ecosystem thinking — not altruistic, just no perceived benefit.
Many ideas are valuable but an OpenAI wrapper — they could crush you anytime. Some easily replicable by open source community within a year if you think about it.
Avoid these areas.
I like ideas with tech or data moats. Hard to find but exist.
5. What are you really competing on? Is it just who has more money or data?
Many areas clearly compete on money or data. Avoid — you’ll never beat Google, OpenAI, ByteDance on money, or Tencent, Meta on data.
AI is long-term. Short term wins are meaningless.
Sometimes I suspect big tech deliberately leaves openings for open source to experiment for free and find best methods, then throws money to dominate. Game over.
No need to compete on money or data. Many other opportunities, no need to compete with big tech directly.
Those are some of my principles. What do you think, what are yours? Welcome discussion.